The fourth industrial revolution is well under way, and expectations are sky-high that it will transform the way manufacturing will be done in the future. Much of the potential lies in the increasing ability to leverage data to significantly improve manufacturing processes. Manufacturers who do so effectively can derive tremendous competitive advantage from it, with up to 20% conversion-cost savings. But despite this tantalizing reward, most analytics efforts within the industry fail to deliver anything close to that level of saving. One of the reasons they fall short is because they do not target high-value opportunities. Where, then, are the big opportunities to use analytics to transform manufacturing?
This article is addressed to data scientists and industry professionals alike — to those who want to understand the diverse opportunities and savings available through the use of manufacturing analytics. What we will discuss draws upon our experience bringing data science and analytics to BCG manufacturing clients. In many cases, these clients already excelled in traditional operations and at implementing lean. Even so, faced with ever-tighter margins and increasing complexity, these companies were looking for the next performance step change through analytics. We describe below how analytics can deliver that performance step change at the core of the manufacturing process; that is to say, on and around the manufacturing line itself. We have deliberately excluded tangential areas such as predictive maintenance, supply chain and warehouse optimization which have already been covered extensively elsewhere.
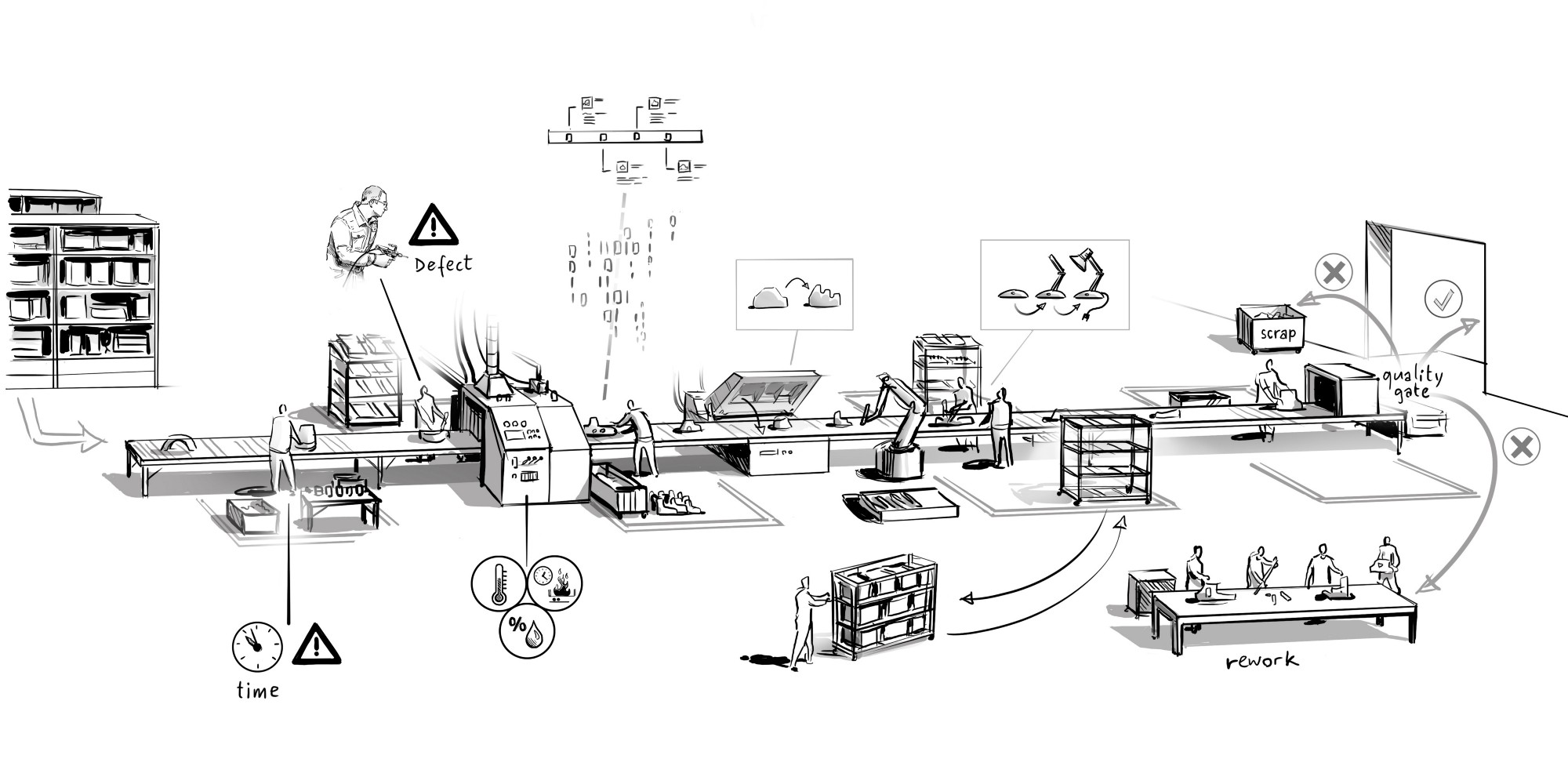
Figure 1: Illustration of a manufacturing line
The Data Foundation for Manufacturing Analytics
Manufacturing lines convert resources such as materials, energy, time as well as machines and workforce into finished products. This conversion takes place either through a sequence of discrete process steps, such as the assembly of parts along stations on the line, or continuously by means of physical-chemical processes, as in the production of pharmaceuticals.
Manufacturing lines can be seen as an implementation of a solution to a complex problem: namely to produce thousands of different products efficiently and reliably on one and the same line. Each product is the result of a series of specific tasks and operations that must be carried out sequentially by machines, by man and, often, by a combination of the two. Each step in the process must be reliably carried out in a precise way and time to meet customer requirements, delivery schedules or industrial quality standards.
For more than a hundred years, manufacturing lines have been setup linearly as a sequence of stations at which workers or machines carry out specific tasks (see Fig. 1) But how good of a solution is the line to the production problem? To find improvement opportunities, manufacturing analytics taps into the high-dimensional space of data from materials, machines, workers and products. This data describes how these resources are utilized and converted step by step into products of certain quality. The ideal data foundation contains the records of all events that take place along the production line, and provides end-to-end traceability, capturing the “when” and “where” and “how” of each and every product that is made. Increasingly, manufacturers collect this data in clouds. More often however, it still resides in separate MES, ERP and quality systems.
Two Classes of Manufacturing Analytics
Analytics generates value only when it changes business decisions or actions. Manufacturing analytics can do this by improving the utilization of critical resources, which, in turn, improves key manufacturing performance metrics such as Operating Equipment Efficiency (OEE) or Right-First-Time (RFT).
There are essentially two classes of manufacturing analytics applications. A first class addresses the process of planning the use of resources such as machines, materials, time and workforce. Here, analytics determine how much of and when each resource will be needed to make a certain quantity of product. This class provides production forecasts and production plans, as well as production schedules and the sequences in which a certain product mix will be made. A second class of applications determines how these resources will be converted into products by maximizing resource-efficiency and product quality.
Specific analytics applications include (see Fig. 2):
1. Production Forecasting and Planning
2. Production Scheduling and Sequencing
3. Line Setup Optimization
4. Line Staffing Optimization
5. Personalized Workforce Upskilling
6. Optimization for Product Quality
7. Yield Maximization
8. Alerts and Previews
Figure 2: Manufacturing analytics application landscape
Class One Applications: Resource Planning
1. Production Forecasting and Planning: The Importance of Accuracy
The first step toward optimizing resource utilization is to know how much of a given resource will be needed. The key to this lies in understanding future customer-product demand and deriving accurate production forecasts. Different products are manufactured in different ways, with different tasks and steps, often with varying degrees of difficulty for the workers. Hence, accurate understanding of the upcoming product mix is fundamental to determine the optimal configuration of the line, and to make the right amount of resources and inventories available at the right time. Many companies continue to rely on best-guess and incomplete manual forecasts from their sales teams, who rarely make demand forecasting a priority task. From the start, this lack of accurate, forward-looking product-mix visibility undermines the ability to configure the manufacturing process for optimal efficiency, throughput, and quality. The ability to accurately forecast demand and production depends to a great degree on the industry and product in question. Applicable analytics methods can range from time-series forecasting to feature-based predictions. Which one is best suited depends to a large extent on the time horizon and context.
For short-term forecasting of product demand, ARIMA and related models such as UCM can often provide a good starting point (The article “The complex challenge of demand forecasting” elaborates further on the methodology).
A leading global cement manufacturer used to rely on manually created demand forecasts its salesforce would periodically generate by calling hundreds of customers and asking them for their upcoming needs in terms of tons of cement. This data would then be manually aggregated and split down by cement plant to deliver the foundation for plant production plans. This process, in addition to tying up significant resources, proved to be highly inaccurate and resulted in cement plants incurring multimillion losses a year. Cement demand follows strong cyclic patterns, with short-term correlations and dependence on external factors such as rain fall, temperature and GDP. Modelling these factors in an ARIMA-type statistical forecast model has improved the forecast accuracy on more than 90% of all cement types. At the same time, it has decreased forecasting errors by more than half, achieving greater than 90% accuracy on large volume products. For this client, the use of analytics improved production and energy planning, and enabled it to accurately schedule yearly maintenance shutdowns into low-demand time windows. The resulting benefits added up to €800K per plant and year. Furthermore, these statistical forecasts established a reliable single-point of truth, accepted across the commercial and production sides of the organization and with consistent stakeholder-centric demand-views, including by plant, sales representative, area, region, and country. These statistical-demand forecasts therefore have also improved the overall Sales & Operations Planning process.
2. Production Scheduling and Sequencing: Minimizing Changeovers
Switching production from one product to another, known in the industry as making a “changeover”, can involve the time- and resource-consuming exchange or re-configuration of machines, tools and production lines. This unproductive time can account for several percentage points of reduced machine utilization and line downtime. In non-optimized operations, as much as 50% more changeovers can take place than necessary, representing a staggering loss in productivity.
Manufacturing analytics can minimize these efficiency losses by helping to optimally schedule and sequence the production of different products. The goal is to minimize the number of unnecessary changeovers, taking into consideration factors such as product demand, inventory levels, and availability of resources. The schedule and sequence optimization then frequently falls into the well-studied class of computer science and operations-research problems known as the “Job Shop Problem” or, more generally, “Resource Constrained Scheduling Problems”.
The pressing facility of a parts supplier can produce more than 400 different parts. While every part requires a specific press in order to deliver the desired part shape, the production line can carry only 40 presses at a time. The product mix ordered by the customer, on the other hand, can vary a great deal more. The manufacturing challenge is to configure the line correspondingly and dynamically to deliver the ordered products in the most efficient way possible. Replacing a press takes about 3 minutes and can take place as many as 30 times a day. Hence, the schedule for each press, which defines when and where each press is placed on the line and when it is taken off the line and replaced, must meet customer demand — while minimizing the number of times the line needs to be stopped to replace one press with another. Given the combinatorial complexity of the task, it should come as no surprise that the manual press-schedule developed by the client required twice as many change-overs than the schedule optimized using manufacturing analytics, which delivers 1% less line downtime.
Class Two Applications: Optimizing resource conversion
3. Line Setup Optimization: Removing systematic bottlenecks
Frequently the line setup, which defines the work and tasks allocated to each station, is not well adapted to the actual mix of products the line has to produce. This leads to systematic, “built-in” structural line inefficiencies and bottlenecks that prevent workers from completing their tasks at their stations “in-takt.” These bottlenecks, in turn, force downstream workers to wait for upstream tasks to be finished before they can proceed.
Analytics can help optimally balance a line by simulating both the dynamics on the line and individual stations as a function of incoming product mix and sequence. Discrete-event simulation and Monte Carlo methods can evaluate different line-setups and provide both tactical information, such as where and when bottlenecks on the line occur, and strategic information, such as whether a fixed conveyor, a decoupled conveyor, or even a network-like arrangement of flexible manufacturing cells (FMCs) is the better option. With this knowledge in hand, managers can understand the drawbacks and benefits of different options, and setup the line correspondingly. This might involve removing built-in bottlenecks by rebalancing workload, or making a greenfield project decide between conveyor and FMCs.
A recent BCG study (“Will Flexible-Cell Manufacturing Revolutionize Carmaking” https://www.bcg.com/publications/2018/flexible-cell-manufacturing-revolutionize-carmaking.aspx) showed by means of simulation that, particularly in the presence of high product variety and complexity, Flexible Manufacturing Cells can yield as much as a 12% increase in worker utilization, and significantly higher throughput compared to conveyor belts. On conveyor belt lines, for example, workers frequently wait for cars to arrive from upstream stations. With FMCs, it is the parts that wait for the workers to become available. This is because longer process times at one FMC do not slow down or stop the entire assembly process at other FMCs. These situations are unavoidable with conveyor belt lines due to their rigid linear setup.
4. Line Staffing Optimization: Leveraging Worker Skill Profiles
Across industries, manufacturing requires manual completion of specific tasks, especially in discrete assembly. Each worker has different skills to carry out certain tasks, derived from his or her experience. Some workers are faster and more reliable, while others tend to have a higher rate of line-stoppages or defects depending on the product and station which they are working at.
To turn these variations into an advantage, managers must first recognize the high degree of diversity between products, tasks, and skills, in particular when product mix varies over short time scales. With this knowledge, managers are able to allocate workers strategically to tasks and stations. More often than not, though, managers base these line staffing decisions on other criteria, and rarely on actual performance data, as they should.
One client’s car seats factory had a five-fold variation in worker performance on the same tasks, and line-efficiency and defect rates that were highly dependent on seat-mix and worker-station allocation. Predictive models were trained on historic data from defects, line-stops, seats and staffing configurations to calculate the probability of line-stoppages and defects for a given staffing configuration and incoming seat-mix. By simulating different staffing configurations, the “best-fit configuration” for each shift was determined, which the shift-managers could use during the shift briefings to advise workers on their tasks. Use of the configuration has reduced line-stoppages caused by workers by a full 50%.
5. Personalized Workforce Upskilling: Enhancing Training Effectiveness
The data used for staffing optimization can also serve as a foundation to identify specific workers’ weaknesses and pair them with appropriate training. Compare this to the norm, in which training needs are usually discovered on an ad-hoc basis. Assignment of training usually comes down to a manager’s ability to identify problems on the line, even as he or she deals with other pressing issues. The inability to pinpoint the need for training not only harms efficiency and quality, but reduces management’s ability to leverage training and performance as incentives for workers.
Using the line’s production data and shift plans, manufacturing analytics can help determine an individual worker’s contribution to quality and efficiency while he or she is performing certain tasks on certain products. This insight provides very specific and individualized training opportunities. (Note: The ability to take full advantage of the data to help specific workers improve their skills depends on a company’s ability to tie performance data to individual workers. Such use of personalized data is typically determined by legislation.)
6. Optimization for Product Quality: Defects Drain Resources
Delivering quality throughout the manufacturing process is equally as important as efficiently using resources. In some factories we have seen, the rate of defective parts was so high — as much as 30% — that the factory spent almost as much money making defective products as it did making quality products. The factories would then have to commit even more resources to repair or scrap these defects. Clearly, understanding and correcting the causes behind defective parts can provide significant cost savings.
Especially in the process industry, resources undergo complex physical-chemical processes that determine the physical properties and quality of the finished goods. In practice, despite the complexity of these processes, the production parameters (“recipes”) that define how these resources are used are often determined manually over many months by trial and error. Determining these recipes normally relies heavily on expert knowledge. Even so, the resulting recipes are rarely optimal.
By combining the recipes of different products and their resulting quality and defect rates, manufacturing analytics can improve quality delivery. The first step is to develop a model that predicts how the quality of the finished product depends on the parameters of production — that is to say, on the recipe. Once this model is in place, methods such as simulated annealing can help find the best parameter combination that minimizes the defect rate. This process can help define new and improved set-points for production, and massively shorten the industrialization phase.
A global PU player client makes foam parts by injecting a mixture of different polymers into a mold. Each of the more than 350 part-types has a specific injection recipe that determines, for each of 6 polymer components, the proper shot duration, flow speed, temperature, pressure and other physical parameters of the injection and mixing process. Overall, however, approximately 25% of the parts produced have at least one defect, such as air pockets, that requires the part to be either reworked or scrapped. Using a gradient-boosting algorithm that was trained on more than 1.5 million parts, the client is now able to predict the defect rate for a given part and a given recipe. By searching the parameter space for those parameters that minimize defects, the quality manager can now identify better recipes. This revealed an overall defect-reduction potential of one third.
7. Yield Maximization: More with less
Many industries require large amounts of energy and other expensive raw materials. A few percentage points worth of consumption savings on these resources can translate into multi-million-dollar PnL savings for a single plant or plant network. Despite the complexity of having to configure more than 20 parameters on large machinery, the critical operating set-points that determine consumption are still often based exclusively on expert knowledge.
Predictive analytics can inform on the hypersurface of potential set-points and their energy consumption and quality. In fact, frequently solutions exist which lead to a similar outcome, but require different amounts of resources. This also applies, for example, to the rate of wear of spare-parts. Machine operators can use this information to make better decisions on set-points and maximize the yield from precious resources.
An Asian copper manufacturer we have worked with was struggling with declining copper yields in its smelters. The company’s converting furnaces are fed melted copper matte, which contains mostly copper and iron sulfide. By blowing oxygen through the matte, the furnace converts it into slag and blister, which contains copper and sulfur dioxide. Ideally, all the copper is contained in the blister. The client however, was losing a significant amount of the copper (2%) into the slag. The entire process involves more than 100 parameters including raw material mix, oxygen injection, and other factors. The company’s engineers had not been successful at modelling the process to explain — and avoid — the loss. A neural network, in turn, successfully integrated the dependencies between parameters of the process to predict copper loss. By simulating multiple parameter settings on the neural network, the client was able to determine better operating set-points which reduced the loss almost entirely — and to experience a 2% increase in revenue and 1% increase in profit.
8. Alerts and Previews: Using History to Predict and Prevent Risk
Beyond strategic applications for analytics, there are also powerful tactical applications. For example, predictive risk alerts can help mitigate short-term efficiency risks that can account for up to 30% of efficiency losses. These alerts can help avoid unexpected line stoppages or delays in task-completions by workers. They can also reduce quality risks by alerting managers and workers to specific events, such as when a sequence of particularly complex products comes on the line.
Analytics can help managers understand which production patterns correlate with efficiency drops or defects, and predict the probability at which either of these will happen. By combining the configuration state of the line with a production forecast, these probabilities become forward looking. When, for example, the defect probability for a certain product at a particular station and time in the future exceeds a threshold, a predictive alert can be elevated to operators or line managers. Visualizing these alerts and probabilities forward over time is a powerful way to preview when and at which station on the line risks will appear. With this forewarning, shift teams have the ability to prepare for — and prevent — these risks.
The factories of our leading car-seat manufacturing client can produce more than 2,000 seat variants on the same line. When the factory’s customer, a car maker, sends a seat order to the factory, the factory will produce these seats just-in-time over the next 240 minutes. This puts enormous pressure on quality and efficiency, as penalties for delays or inferior quality are high. Working with the client, we built a predictive alert system to provide a production-risk preview and advise shift and line managers about which seat at which station and at what time will have a high risk of line stoppage or defect. The model is a Random Forest and combines current worker-station allocation, more than 300 features per seat, and the incoming seat order. By continuously displaying its output above the production line, the model helps managers anticipate and mitigate these incoming risks.
Get Analytics in Place and Manage the Change
The opportunity to fully leverage manufacturing data and analytics is within the grasp of many companies. It comes closer still with increasingly inexpensive and accessible Internet of Things (IoT) devices. With this technology so readily available, many BCG clients now seek advice on how to fully exploit it.
The above-mentioned solutions are good starting points for the ideation and prioritization of high-impact analytics applications. While the examples are neither exhaustive nor applicable in each and every manufacturing context, they outline some of the most promising areas for the use of analytics to deliver high value.
The details of a manufacturing analytics application will always depend on the specific industry, product and process involved — and, often, on the individual factory itself. No off-the-shelf solution could deliver this level of customization. Even when companies focus on the right high-value opportunities, though, key challenges will remain. Data quality, richness and accessibility, for instance, are typically low in manufacturing environments that previously used data for reporting purposes alone. And with the introduction of analytics tools, companies must learn to actively manage the cultural and organizational changes that follow.
At BCG Gamma, we are convinced that high-value transformative analytics can be delivered only through a combination of state-of-the-art data science and a deep understanding of the industry. To that end, we deliver bespoke solutions tailored to each client’s specific operations, along with a clear change agenda. By bringing together best-in-class data scientists, generalist consultants, and operations and industry experts, we can show you how to use manufacturing analytics to increase your production efficiency, enhance your product quality and, ultimately, improve your bottom line.
Figure 2: Manufacturing analytics application landscape